Proof-of-Concept at large insurance company in Italy results in significant performance improvement over earlier methods
March 8, 2021
LARUS Business Automation Srl
Venice Italy, March 8, 2021: LARUS Business Automation today announced results of the application of the LARUS Galileo Graph Explainable Artificial Intelligence (GXAI) platform powered by Fujitsu Deep Tensor® Technology at a large insurance company in Italy. Based on the preliminary results, the fraud detection rate improved from 18% to 81% while the number of false positives decreased from 82% to 19% over traditional rules based approaches. A demonstration of this solution will be shown at the AI and Big Data Expo Conference being held on March 17 and 18, 2021.
In Italy, according to the Institute for the Supervision of Insurance (IVASS), in 2018 there were 42 million insured vehicles, nearly 3 million claims of which more than 600 thousand were potential cases of fraud. The size and scope of the problem puts an enormous burden on human analysts requiring automated solutions to address the problem. Using the platform, LARUS was able to automate a significant portion of the analysis process leading to improved efficiencies and reduction in need for cumbersome manual analysis.
“With our solution developed in collaboration with Fujitsu, the insurance company reduced the number of false positives and, in doing so, could redirect valuable investigative resources to critical areas of the business” said Lorenzo Speranzoni, CEO at LARUS. “We are looking forward to applying this solution to other vertical industries where GXAI can have a significant financial and social impact”.
“Fujitsu Deep Tensor technology was a key advancement in Graph XAI research offering unparalleled insights into a wide array of application datasets” said Seishi Okamoto, Head of AI Research at Fujitsu Laboratories Ltd. “Through our collaboration with LARUS we have demonstrated how such innovative technologies can be applied to support enterprises in their digital business transformation journey”.
Background
The heterogeneity of insurance data makes it difficult to identify fraud as the underlying information is very complex and the data must be presented in a certain way to it. It is also imperative to target the right claims, at the right time. Examples of common false claim schemes include falsehoods about identity and location – when people use someone else’s address to register and insure their cars because they know insurance rates are lower in that area, or fictitious infringements in road accidents and exchanges of roles in claims – when the subjects of claims are recurring over time but in different roles.
Solution Overview
Existing fraud detection applications use a claim-centric approach but it is more difficult to discover claims involving groups of subjects rather than single individuals. To address this problem, Galileo XAI uses an integrated and unified graph database whose nodes represent vehicles, claims and subjects and edges represent the relationships between them. The graph DB in the tested solution contained about 16 Million nodes and nearly 21 Million relationships.
Harnessing the graph database, it is possible to define classes of suspicious patterns – called rules, (expressed as cypher queries) – based both on topological properties and on node/edge attributes. These rules generate alerts that the insurance company can analyze and determine whether it actually represents fraudulent behavior or whether it is a false positive.

Images 1-2: improvement of fraud detection rate and decrease of false positives
Rules are inherently not static and need to evolve with the dynamic nature of fraudsters. Using the graph topology in combination with Deep Tensor lets the analyst uncover new frauds and new behaviors, LARUS was able to automate a significant portion of the analysis process leading to improved efficiencies and reduction in need for cumbersome manual analysis.
Together with the pattern-matching features, Galileo XAI provides a visual tool to easily analyze the alerts found by the rules, allowing interaction with those subgraphs that match a desired pattern, in order to set the outcome of the investigation. By exploiting the connectedness of data and extracting new indicators based on the structure of the graph, the solution enabled the anti-fraud team to focus only on relevant groups of subjects or accidents reducing the set of false positives. Furthermore, these indicators are seamlessly used by Deep Tensor to constantly improve the results. There are 3 suspicious subjects who have a high probability of being fraudsters (the 3 red subjects in the middle of each community). The red neighbours give us a clear picture of their contribution in classifying the 3 subjects.
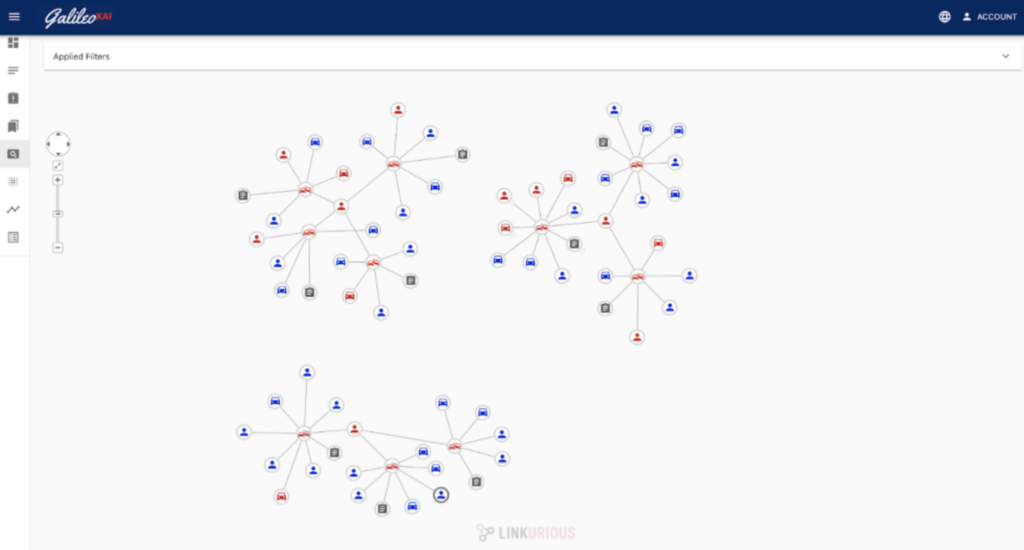
Image 3: there are 3 suspicious subjects who have a high probability of being fraudsters (the 3 red subjects in the middle of each community). The red neighbours give us a clear picture of their contribution in classifying the 3 subjects.
Finally, when AI algorithms may have a direct impact on human beings it is essential to give a clear explanation of the results as required by new laws including GDPR. Galileo XAI with its powerful graph visualization takes the explainability outputs given by Deep Tensor and shows them in a human understandable and friendly format for quick consumption thereby satisfying a core requirement for the adoption of AI systems.
Press Contacts
LARUS Business Automation S.r.l.
E-mail: [email protected]
About Larus
Headquartered in Venice, Italy, LARUS helps companies around the world designing large-scale data-driven platforms based on the latest innovative technologies. As a world-wide top “Premier Solution Partner” of Neo4j, its “Certified Professionals” have been helping universities, institutions and companies succeed with the world’s leading graph database since a decade. LARUS has a wide experience in different domains from government, insurance, financial institutions to manufacturing, retail and telecom with a strong track record and its R&D department works with many universities on several topics from graph data-visualization to graph algorithms and machine learning in order to validate their application to customers’ use cases.